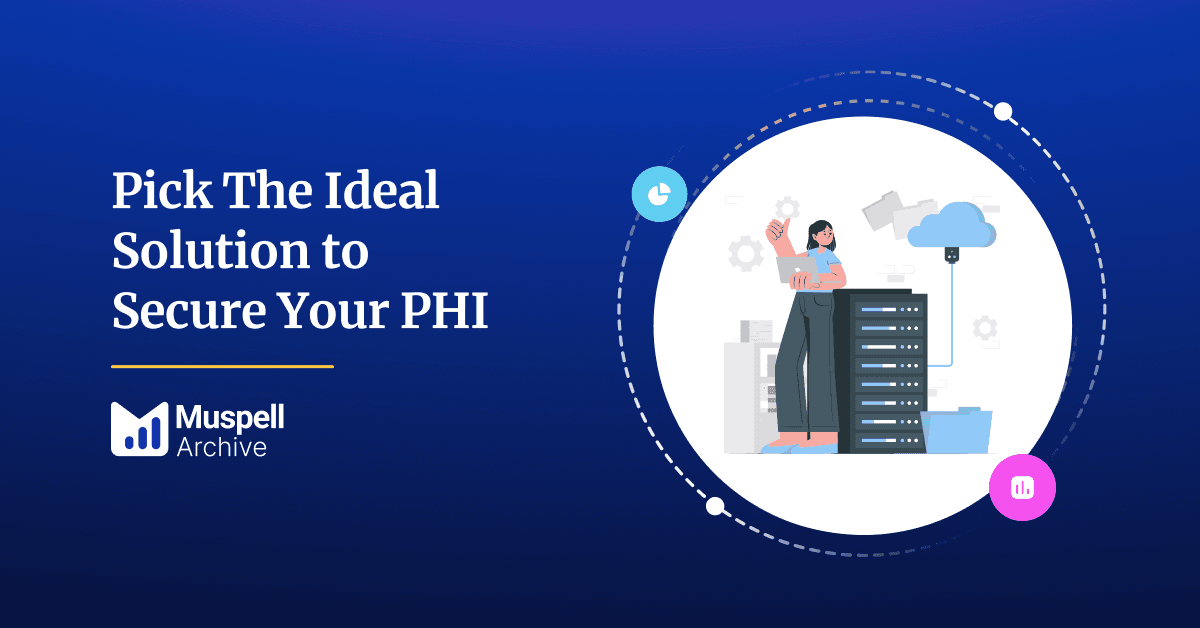
Choosing Your Ideal Healthcare Data Archival Solution
Since the release of the Cures Act Final Rule in May 2020, the US healthcare industry has been experiencing a …
When health systems broach the task of converting data for the purposes of implementing a new EHR such as Epic, it’s critical to perform as close to an apples-to-apples comparison of PHI as possible. While the hospital IT team may have a firm grasp of how to transition between interoperability standards to physically move the data from Point A to Point B, the technical team should never be the final word on whether EHR data conversion was truly a success for supporting physicians at the point-of-care. Clinical users are naturally more adept at deciphering the medical information they’re looking for within an electronic health record, and can typically spot rather quickly when data doesn’t look accurate or whey key data points are missing.
Today we’ll focus on a few key evaluation points that demonstrate why clinical validation for EHR data conversion is so critical, and how it can support successful adoption of Epic.
One of the most important aspects of data conversation for Epic implementation is either matching or improving upon the quantity of structured data. Many of the promises of Big Data in healthcare and other analytics opportunities are realized only when data is collected and captured in a structured manner, serving it up for BI and analytics tools to accurately leverage. So when clinical users are reviewing conversion data, it’s important to evaluate the volume of data that is being stored in a structured format.
For example, if lab reports were previously stored and displayed as PDF (read-only) reports, converting to parsed, structured lab data in Epic would be a huge value add to be trend values and even power Clinical Decision Support (CDS) tools. But this value-add is only half as cool if the ranges and values aren’t being stored correctly. Bringing in a medical professional to confirm both the format and the values are as expected (or better), will make the data conversion efforts far more fruitful.
Building upon the lab results example from above, the way in which values from multiple sources are normalized (or not) during data conversation is a big deal for driving optimization and better utilizing some of the savvy tools within EHRs like Epic. If in the process of data conversion, test identifiers are dropped/lost that tie results to a consistent value range, for example, an abnormal flag could be thrown for the exact same value deemed normal by another lab. This normalization also applies to units and measurements (such as when offices report height by inches or a combination of feet and inches). If the conversation methodology doesn’t account for this, these are the types of defects that clinical validation will flag for some “data massaging.” (This is where an integration engine may be needed to support normalization and data translation for EHR conversion efforts.)
If a busy clinical end user has to poke around endlessly in their Epic instance to locate data for a patient, there is a good chance that they may miss that data and/or grow increasingly frustrated with the technology. Sure, EHR training for providers goes a long way on this front, but so does tapping into the wisdom of a clinical user for UAT of data conversion between EHRs. A key component of establishing usability is, as they say, “Location, location, location!” Does all of the immunization data show in the immunization section? Are problems reported in a consolidated view, in a single code format for consistency? Are radiology reports and lab reports in relevant, unique tabs? Especially with unstructured reports, which continue to be an element of the interoperability equation whether we like it or not, a user having to look in a half dozen locations to find a needed document is not going to drive the ROIs associated with a sophisticated EHR like Epic.
While they may not be able to define the how, clinical analysts can help Epic implementation and data conversion teams better define how integrations should be organized, tagged, and ingested to minimize click-arounds and encourage successful EHR utilization.
Join over 3,200 subscribers and keep up-to-date with the latest innovations & best practices in Healthcare IT.
Since the release of the Cures Act Final Rule in May 2020, the US healthcare industry has been experiencing a …
Healthcare has witnessed a significant increase in Mergers and Acquisitions (M&As) in the last decade. One …
An authorization for Release of Information form (ROI) allows your patients to specify which parts of their …