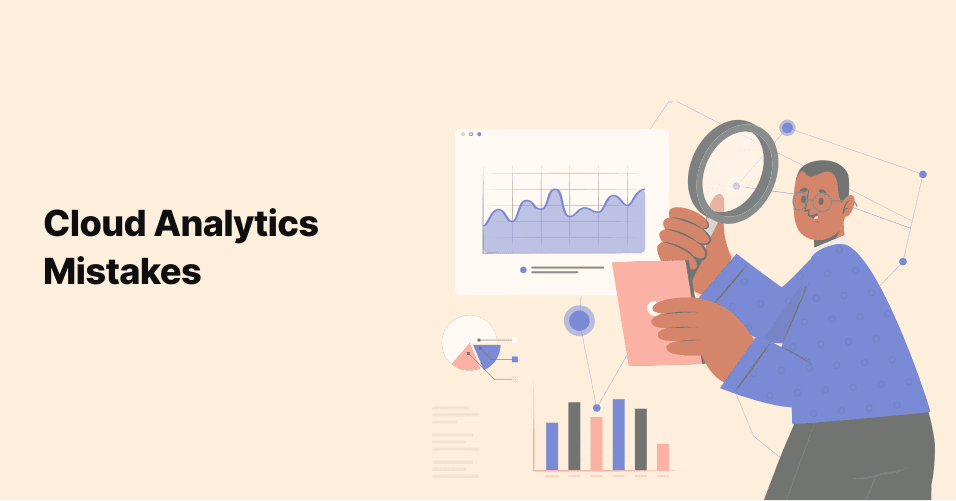
Healthcare Cloud Analytics Mistakes To Avoid
Data-driven decisions are the way of the future. Not only is there wisdom in using facts versus assumptions to …
In the digital era, healthcare organizations must effectively harness extensive data to enhance patient care, streamline operations, and drive product and service innovation. Despite technological advancements, many struggle to integrate diverse data sources and optimize Business Intelligence (BI) applications effectively.
The Healthcare Information and Management Systems Society (HIMSS) offers a structured roadmap—the Adoption Model for Analytics Maturity (AMAM)—guiding healthcare providers towards comprehensive data maturity.
This article delves into the HIMSS AMAM, detailing how healthcare providers can partner with data service experts to accelerate their journey toward analytics maturity.
Explore:
Data maturity refers to an organization’s ability to perfect its analytics process, optimizing every step in the data cycle.
Before the HIMSS model for analytics maturity, frameworks like the 2002 Sander’s Hierarchy of Analytics Needs and the 2013 Healthcare Analytics Adoption Model attempted to guide health systems through achieving data maturity. These earlier models laid the groundwork, addressing challenges posed by Machine Learning (ML) and automation.
What sets the HIMSS model apart is its holistic approach. Instead of solely focusing on data, it balances data, resources, and processes. This model not only enhances care effectiveness and patient outcomes but also focuses on optimizing financial performance and reducing operational costs, crucial for the fiscal health of any healthcare organization. The engagement of key clinical & financial stakeholders and executives in the data maturity process will align analytics initiatives with the broader business objectives of the organization.
In 2015, the Healthcare Information and Management Systems Society (HIMSS) introduced the Adoption Model for Analytics Maturity (AMAM) to standardize analytics maturity in healthcare.
This model helps health organizations build strong analytics frameworks that benefit patients and medical staff by measuring capabilities from technology installations and processes.
The AMAM has eight stages - from zero to seven. Stage Zero begins the analytics journey, with subsequent stages focusing on data quality, governance, and alignment with the data strategy. By Stage Four, organizations measure and manage evidence-based care, and the final stages enhance care quality through predictive and prescriptive analytics.
Implementing the AMAM often involves developing multiple stages simultaneously. With the right tools and expert guidance, advancing through the HIMSS model can improve healthcare delivery, reduce costs, and decrease hospital-acquired conditions.
The HIMSS Adoption Model for Analytics Maturity (AMAM) framework outlines a comprehensive pathway for organizations to develop their analytics capabilities, progressing through seven distinct stages.
Each stage represents a step forward in the organization's ability to leverage data and analytics to improve decision-making, operations, and outcomes. Let's explore these stages in detail:
1. Stage 0: Fragmented Point Solutions
At Stage 0, organizations start their analytics journey with isolated solutions that lack integration, driven by business demands and market pressures. Despite recognizing the importance of data-driven insights for improved decision-making, their analytics tools and processes remain fragmented.
The primary focus at this initial stage is on the need for a more cohesive and integrated approach to analytics. While direct financial benefits are minimal due to the isolation of analytics solutions, the recognition of integration needs lays the groundwork for future enhancements, preparing the organization to streamline processes and reduce inefficiencies, setting the stage for subsequent financial and operational improvements
2. Stage 1: Foundation Building – Data Aggregation and Initial Data Governance
Stage 1 focuses on establishing a foundational analytics strategy by centralizing data management. Organizations begin to store and manage data in a single location, such as an operational data store or data warehouse, enabling historical reference and easier access.
The primary effort involves documenting and executing an initial analytics strategy that consolidates basic data from multiple systems of record. Simultaneously, they develop data governance frameworks to ensure consistent data management across the organization. This centralization of data storage not only reduces the costs associated with maintaining multiple data systems but also enhances data accuracy, leading to fewer decision-making errors and less redundancy. This stage is critical for setting up more reliable financial reporting and effective budget management.
4. Stage 3: Efficient, Consistent, Internal and External Report Production and Agility
This stage is all about standardizing and streamlining report production.
In Stage 3, organizations demonstrate mastery of descriptive reporting across the enterprise. Various departments can effectively manage data, generate reports with minimal effort, and maintain stable and predictable data quality.
Tools and processes are standardized, data warehouse access is well-managed, and the organization can efficiently produce both internal and external reports. The focus is on producing descriptive reports efficiently and ensuring data quality and consistency.
Streamlining report production reduces operational costs by decreasing the time and labor required to generate reports. Improved data quality and consistency also reduce the risk of costly errors and enhance the reliability of financial and operational decision-making.
5. Stage 4: Measuring and Managing Evidence-Based Care, Care Visibility, and Waste Reduction
Stage 3 focuses on the standardization and streamlining of report production across the organization. This stage showcases an enterprise-wide mastery of descriptive reporting, enabling various departments to manage data and generate reports with minimal effort while maintaining stable and predictable data quality. Both tools and processes are standardized, ensuring well-managed access to the data warehouse and efficient production of internal and external reports.
This emphasis on efficient reporting not only reduces operational costs by cutting down the time and labor required for report generation but also minimizes the risk of errors, thereby enhancing the reliability of financial and operational decision-making
6. Stage 5: Enhancing Quality of Care and Population Health and Understanding the Economics of Care
Stage 5 involves using advanced analytics to bolster decision-making at the point of care and manage population health effectively. At this stage, organizations enhance their data governance to align with quality-based performance reporting, providing more profound insights into the economics of care.
They employ predictive and prescriptive analytics to not only improve the quality of care and patient outcomes but also to optimize financial planning. This strategic use of analytics can significantly reduce hospital readmissions and lower long-term healthcare costs by enabling more informed decisions and resource allocations based on the effectiveness of care.
7. Stage 6: Advanced Analytics Implementation
Stage 6 highlights the widespread integration of advanced analytics within the organization, showcasing the mature use of predictive analytics to enhance data content and clinical support.
This stage is characterized by the strategic use of Machine Learning (ML) and Artificial Intelligence (AI) to enhance decision-making processes, allowing the organization to better predict and manage clinical risks. The deployment of AI and ML not only improves clinical outcomes but also optimizes resource utilization, reduces the need for costly interventions, and enhances operational efficiencies, thereby lowering overhead costs.
8. Stage 7: Personalized Medicine and Prescriptive Analytics
Stage 7 is the culmination of the analytics maturity journey, where organizations achieve the highest level of analytics sophistication.
In this final stage, advanced datasets, including genomics and biometrics, are utilized to support personalized medicine. Prescriptive analytics plays a crucial role, allowing for the delivery of tailored healthcare treatments that cater to individual patient needs. This personalized approach not only enhances patient outcomes by reducing the trial-and-error in treatment plans but also optimizes healthcare delivery. By enabling precise treatment regimens it helps prevent the escalation of chronic conditions and reduces long-term healthcare costs.
Achieving accreditation in the HIMSS Adoption Model for Analytics Maturity (AMAM) framework involves a strategic and methodical approach.
Healthcare organizations must assess their current stage, implement best practices for progression, and overcome common challenges to advance through the stages. Here’s a comprehensive guide to navigating this journey.
1. Assessment and Optimization
Tips on how healthcare organizations can assess their current stage in the AMAM framework:
2. Best Practices for Progression
Proven strategies and best practices for each stage to facilitate advancement to the next level:
1. Stage 0 to Stage 1:
2. Stage 1 to Stage 2:
3. Stage 2 to Stage 3:
4. Stage 3 to Stage 4:
5. Stage 4 to Stage 5:
6. Stage 5 to Stage 6:
7. Stage 6 to Stage 7:
In conclusion, the HIMSS AMAM framework equips healthcare organizations with the tools to effectively harness data, driving not only enhanced patient care but also significant financial and operational improvements. By progressing through the AMAM stages—from foundational data aggregation to advanced applications in personalized medicine—organizations develop robust analytics capabilities. This strategic approach ensures continuous improvement in care quality and cost-efficiency, essential for thriving in the dynamic digital healthcare environment.
Join over 3,200 subscribers and keep up-to-date with the latest innovations & best practices in Healthcare IT.
Data-driven decisions are the way of the future. Not only is there wisdom in using facts versus assumptions to …
The venture of implementing cloud analytics is a big one for healthcare organizations. The effort is enormous, …
When it comes to healthcare claims, managing claims from multiple payers can be a daunting task. The …