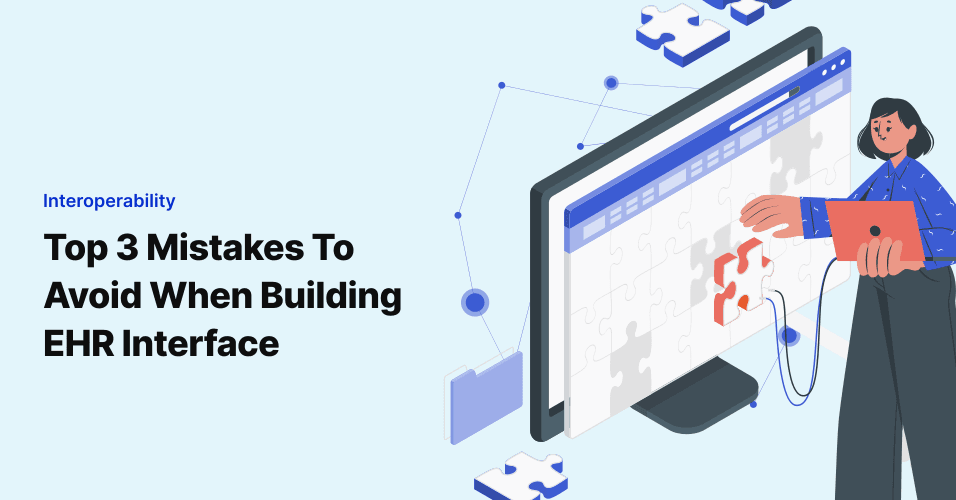
How to Create Healthy EHR Interfaces
One of the key benefits to hospitals for investing in a robust EHR like Epic is to not only cultivate their …
Artificial Intelligence, Machine Learning, and Data Science are the buzzwords-du-jour for healthcare organizations! Every healthcare organization feels pressure to invest in and create AI/ML capabilities and align them to business processes and operations to drive value. However, it is not a good idea to follow the herd when your particular organization’s situation and needs might be different. In this post, I will cover the below:
- What is AI/ML?
- How is Artificial Intelligence(AI) used in Healthcare?
- How is Machine Learning(MI) used in Healthcare?
- How does one decide when a healthcare organization is ready to dive into the deep waters of AI and ML?
Let’s get started with an important question.
Artificial intelligence (AI) is the term applied to characterize a computer system that can mimic human thought and behavior. It is a broad term encompassing older categories like symbolic AI based on logic programming and production rules and newer approaches like Machine Learning. Thus, Machine Learning (ML) is a subset of AI that involves training algorithms to make decisions and predictions related to specific problems and subject areas. Deep Learning (DL) is a subset of ML in which the algorithms used are similar to how the human brain processes data - one such example of an algorithm used in DL is Artificial Neural Networks.
Artificial intelligence is now being widely used in healthcare and the life science industry. In the clinical setting, AI is being used in various ways, including:
Machine learning is a subset of artificial intelligence. Various companies are exploring potential use case of ML in to organize and provide doctors with patient information during telemedicine sessions as well as during virtual visits, and in drug development. The most prominent use cases in healthcare are into automated medical billing, decision support for clinical staff, and in development of robust clinical care guidelines. More are mentioned below:
In this post, I provide some criteria and processes to evaluate if a healthcare organization is ready for AI/ML. I use the acronym CURED for these: Compliance, Use Cases, Revenue vs. Efficiency, Break-Even Point, and Data Maturity.
a. COMPLIANCE:
Are there compliance issues in your organization that you need to find a way to address faster and more effectively? AI solutions can often be the easiest way to address compliance issues, for example, in the way UC Davis Health did: [https://www.healthcarefinancenews.com/news/uc-davis-health-uses-ai-ensure-compliance-patient-privacy-drug-diversion] If your organization has a similar compliance issue that is amenable to an AI solution, that might be an excellent way to introduce AI in the organization.
b. USE CASES:
The task is to identify and document business use cases where AI/ML can derive value. When several business use cases and specifically a few high-impact ones have been identified to which AI and ML can be applied, the task is to quantify the Return-on-Investment for addressing those use-cases. The organization should proceed with implementing AI only if these use cases are substantial enough ROI.
c. REVENUE VS EFFICIENCY:
The third thing to do is to examine if the ROI from the business cases identified is more about increasing revenue or reducing costs. If your ROI calculations for the use of AI are more about increasing the topline than affecting the bottom line, that is an excellent situation to be in. This is not to say that AI/ML doesn’t have a place in improving efficiencies and reducing costs - but the business case is better made if revenue growth is achieved through AI processes.
d. BREAK-EVEN POINT:
The next thing to do is to map out the break-even point. Ideally, it should be less than three years. Three years might seem a long time to wait to reach break-even - but many AI/ML efforts are slow to get off the ground and start delivering value to the business. Therefore, it is important to coordinate with the business early and often to start realizing value. If the breakeven point looks further than 3 years away, it means that the business has not been engaged enough.
e. DATA MATURITY:
The last is to evaluate whether your organization has maturity in both data and the people in charge of managing it. Would you classify yourself as a “sophisticated” organization when it comes to data management and the talent-pool you have in this area? Since data is the foundation of AI and ML, it is imperative for an organization to have a solid foundation of data management and data-quality processes before delving into AI.
So, use these five criteria to evaluate whether your healthcare organization is ready to engage and deploy AI/ML in a meaningful manner.
Join over 3,200 subscribers and keep up-to-date with the latest innovations & best practices in Healthcare IT.
One of the key benefits to hospitals for investing in a robust EHR like Epic is to not only cultivate their …
Operating legacy systems can be a costly endeavor. Maintaining and upkeeping legacy systems require a vast …
Has the idea of switching archival vendors ever crossed your mind as a healthcare provider? For most medical …